Chat With Your Text Documents
Retrieval-augmented generation is a popular approach to quickly build a chatbot using information contained in documents.
Its main purpose is to answer a "knowledge-oriented user queries" which refer to questions or requests from users that specifically seek factual information, expertise, or domain-specific knowledge.
This guide covers the process of setting up and building a RAG Assistant with Rasa using the Enterprise Search policy.
The idea behind RAG is a two step process:
- Use what the user said to query an information retrieval system and fetch relevant documents
- Include the retrieved documents in an LLM prompt, asking the LLM to answer the user's question based on the information provided.
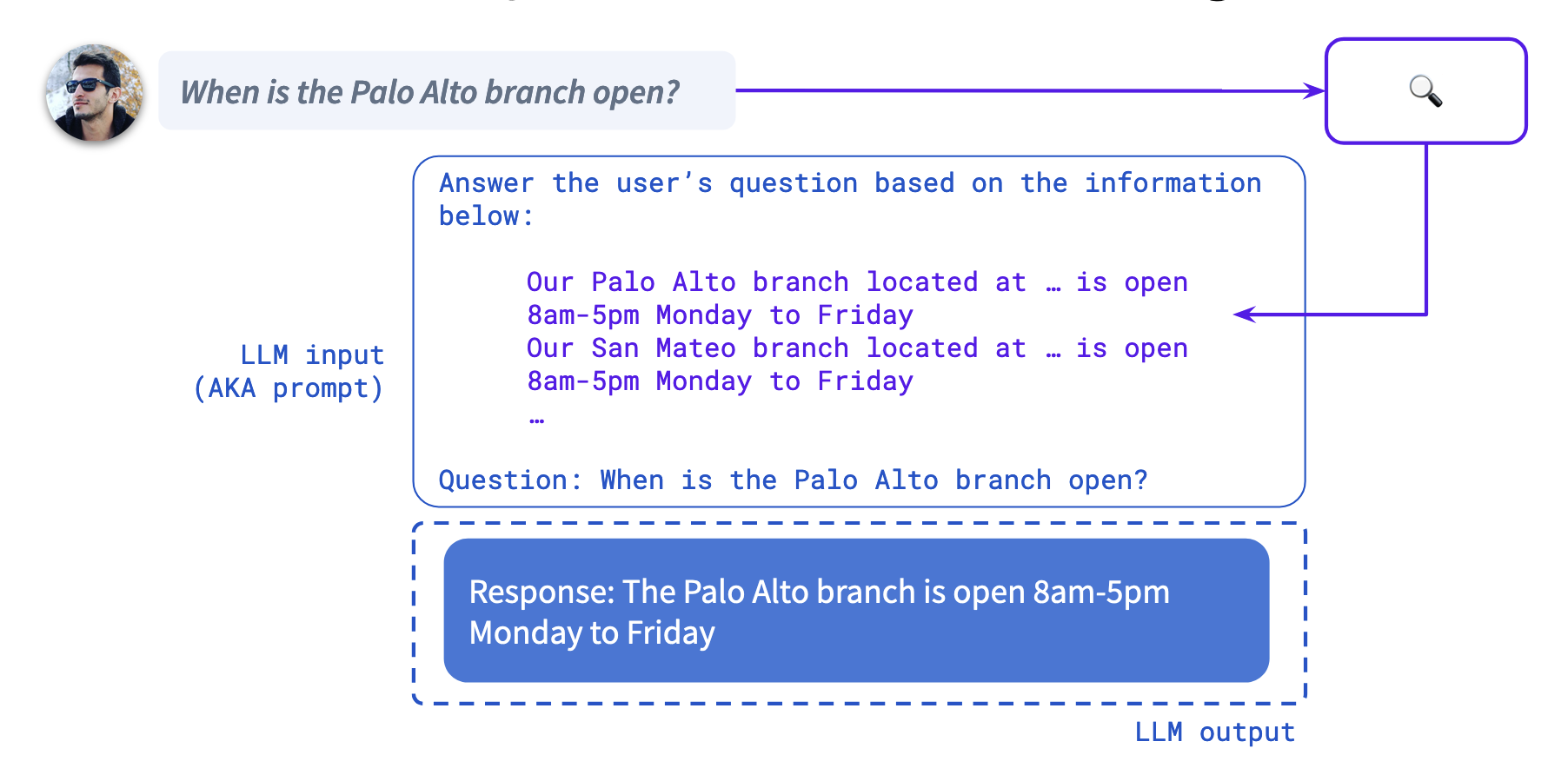
Here are the 3 main steps to go through in order to accomplish our goal
Create a folder called docs/
- Collect your knowledge base/corpus in a text format and dump it into the docs folder: this will represent the source of information from which the LLM will use to fetch context to generate responses.
pattern_search
Override the The pattern pattern_search
defines the assistant behavior for responding to knowledge-oriented user queries.
In flows.yml, add the flow pattern_search
to trigger the default action action_trigger_search
.
The action action_trigger_search
will perform the query and call the LLM to answer the user's question.
Add the Enterprise search policy to your config
By default Enterprise Search Policy uses OpenAI embeddings to embed documents and GPT 3.5 to respond to user queries. You can configure the policy to work with other embedding models and LLMs. Read more here
Below are the default settings:
- Rasa Pro <=3.7.x
- Rasa Pro >=3.8.x
Outcome
Below is a conversation example of how we can leverage Enterprise search using the steps mentioned above.
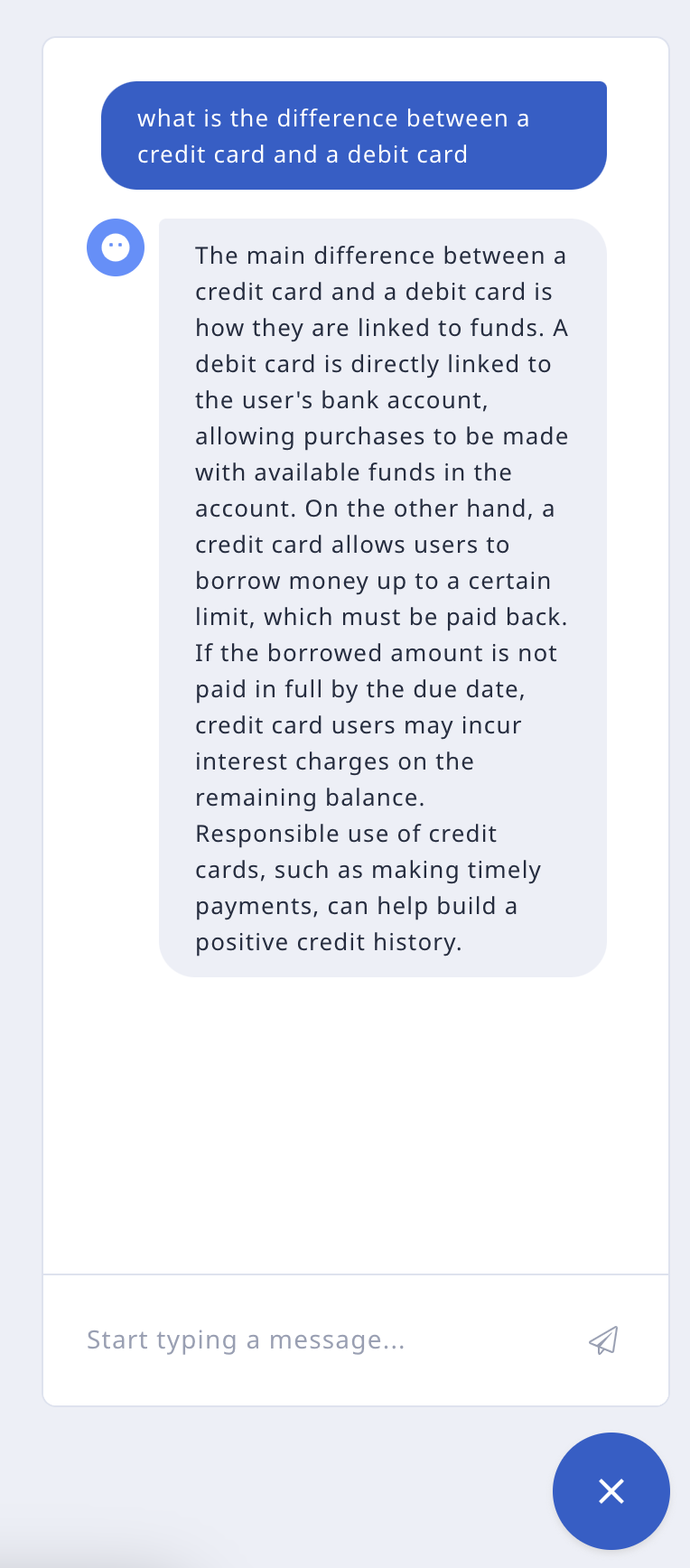
Advanced configuration
The previously outlined setup is designed for initial exploration of the RAG approach with Rasa. The Enterprise Search Policy currently supports Milvus and Qdrant which offer multiple deployment options from self-hosted docker to cloud deployments.
For example, if you want to use the Milvus vector store and embeddings from huggingface, you will have to modify your config.yml and endpoints.yml files.
To configure the EnterpriseSearchPolicy to use these components, add the following lines to your config.yml depending on the version of Rasa Pro you are using:
- Rasa Pro <=3.7.x
- Rasa Pro >=3.8.x
And add the following to your endpoints.yml: